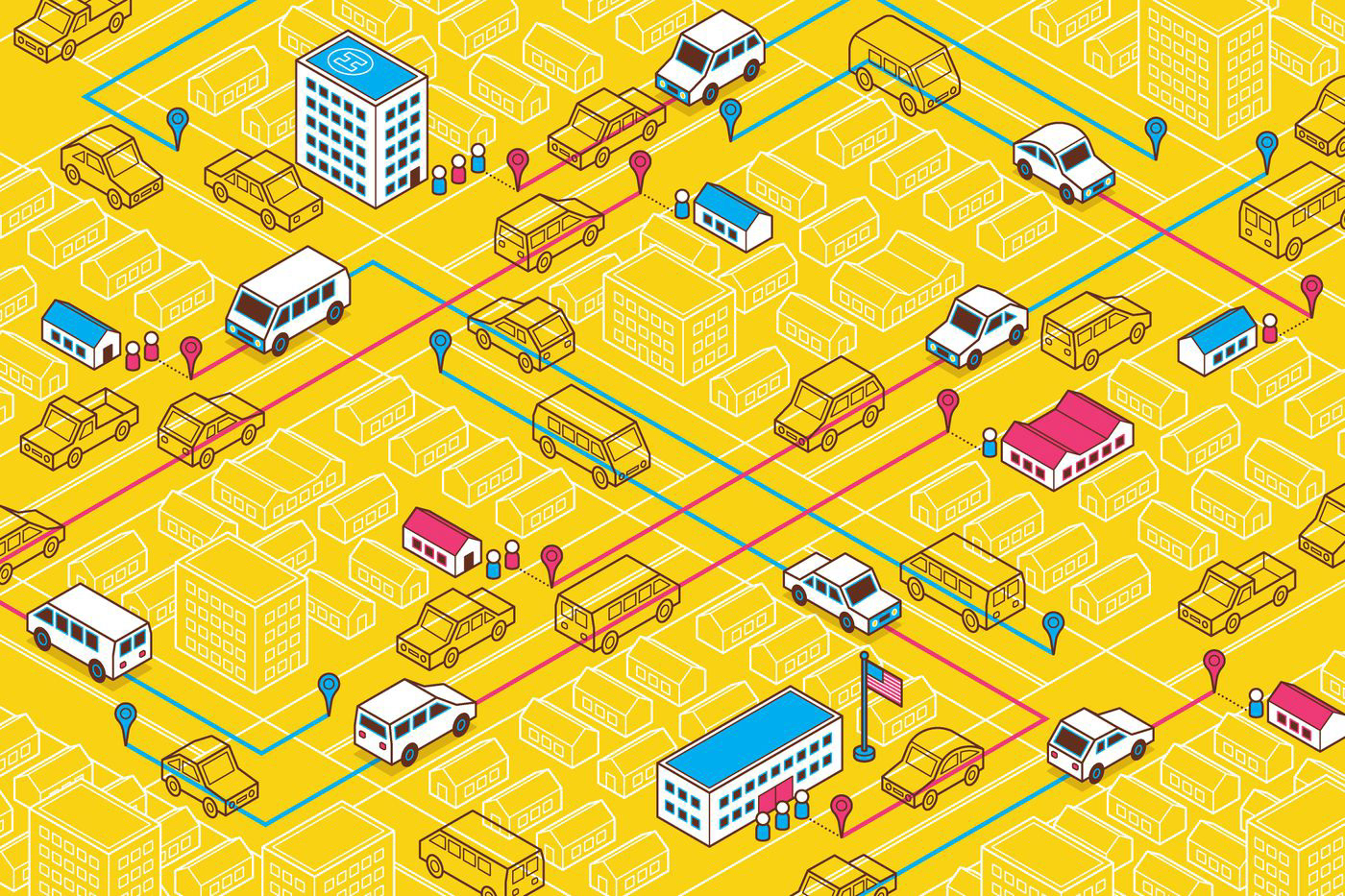
Image source : https://www.theverge.com/2016/9/1/12735666/uber-altamonte-springs-fl-public-transportation-taxi-system
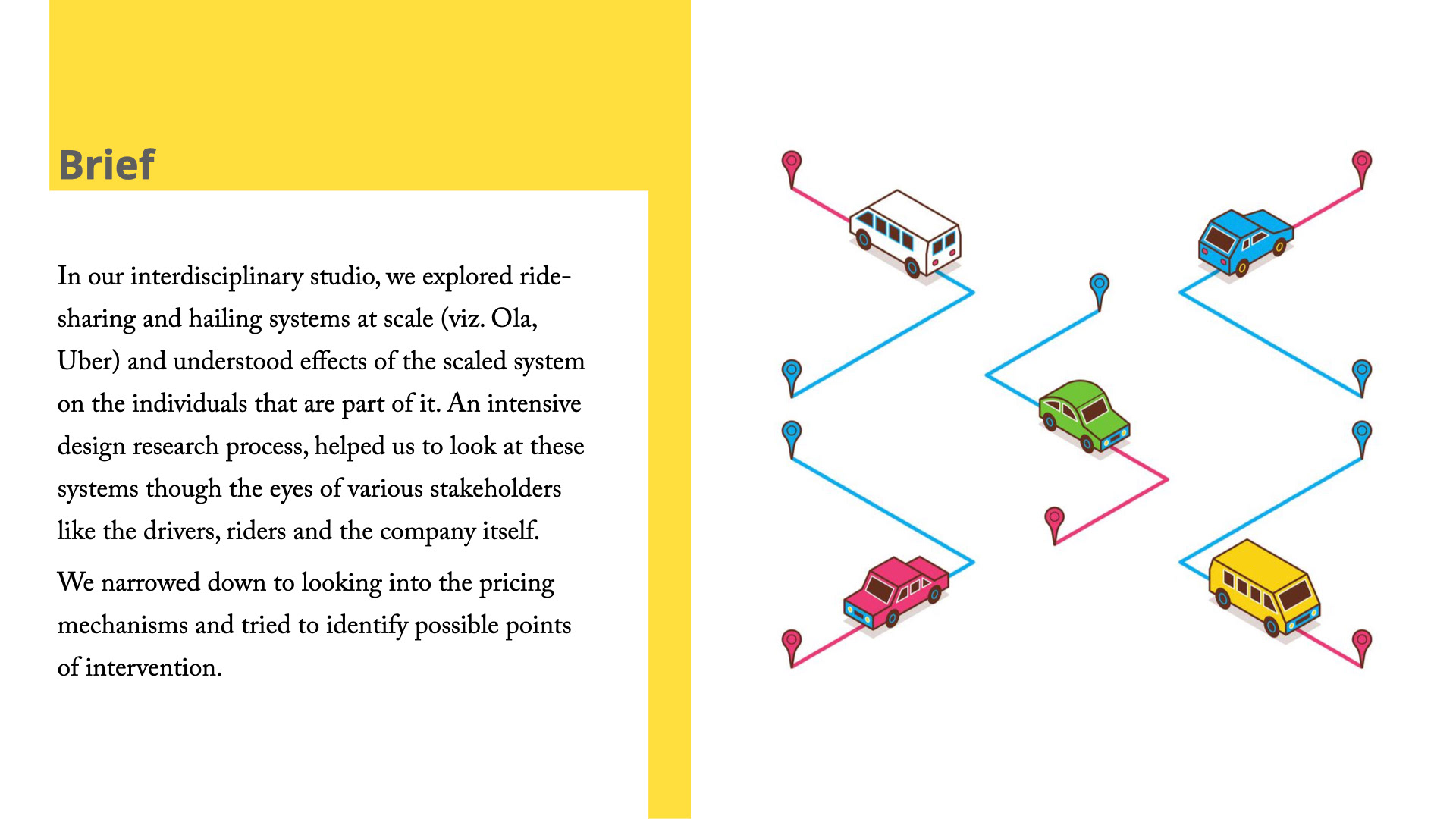
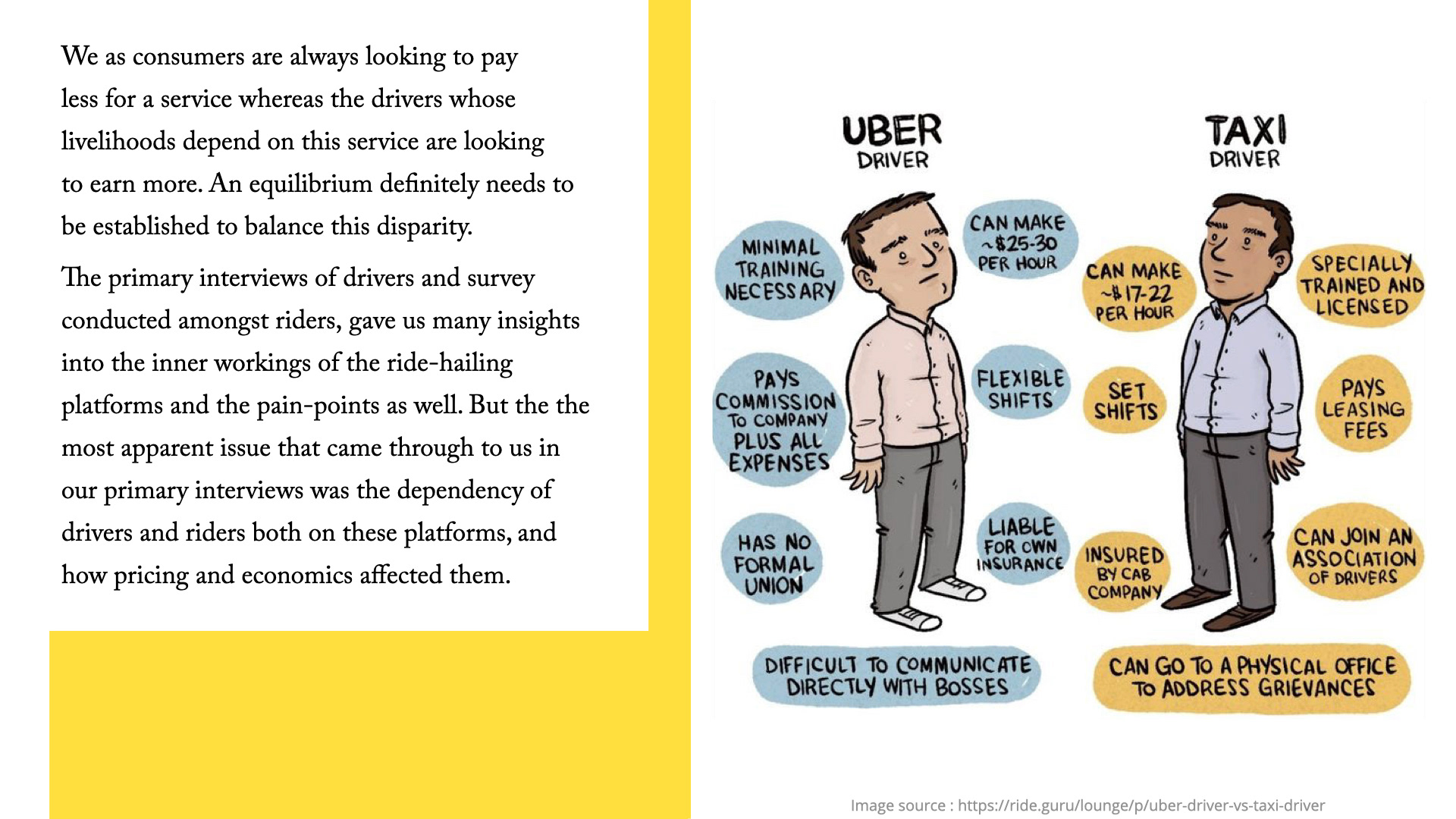
. . .
“We are totally dependent on the platform due to car loans.”
“We don’t get paid to travel to the pick-up point, we pay out of our pockets.”
. . .
Various aspects of Pricing in Ride hailing systems
Before we could jump into “making”, we had to look at the aspects of how pricing works at the moment (of doing this research) in Ola and Uber.
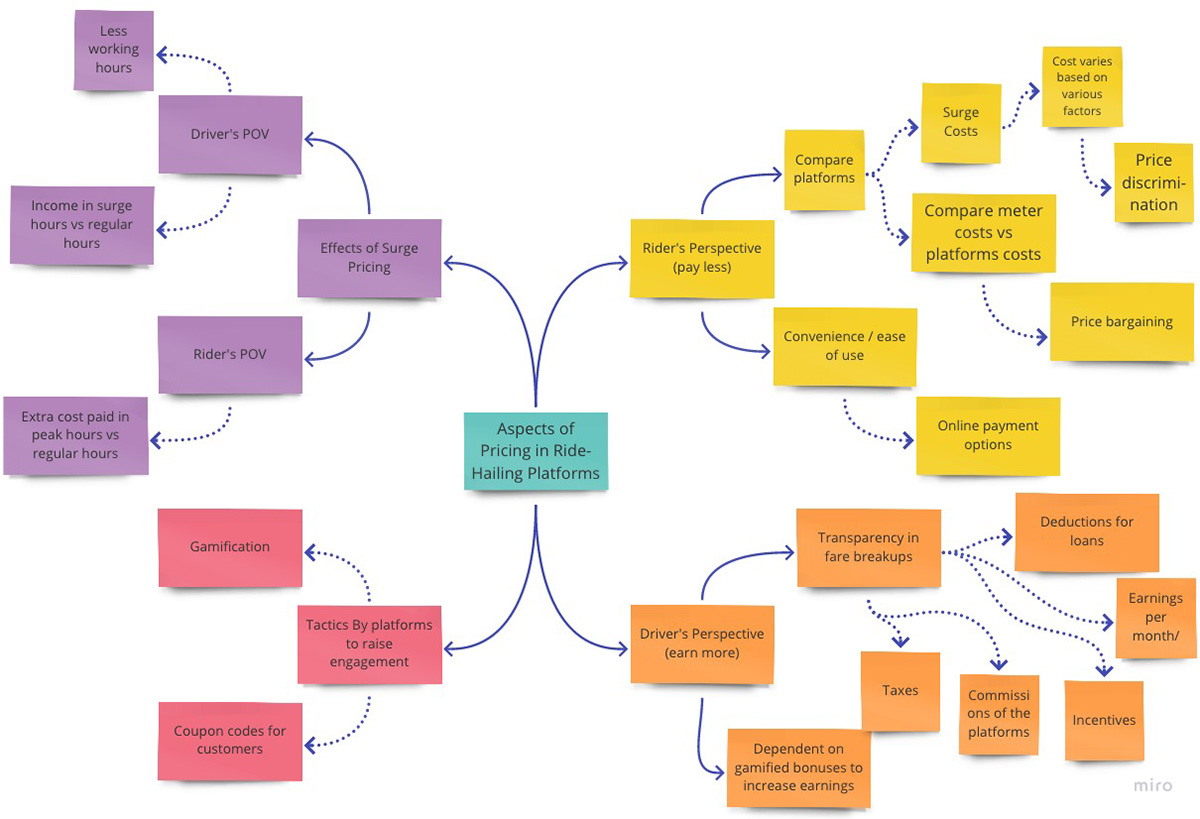

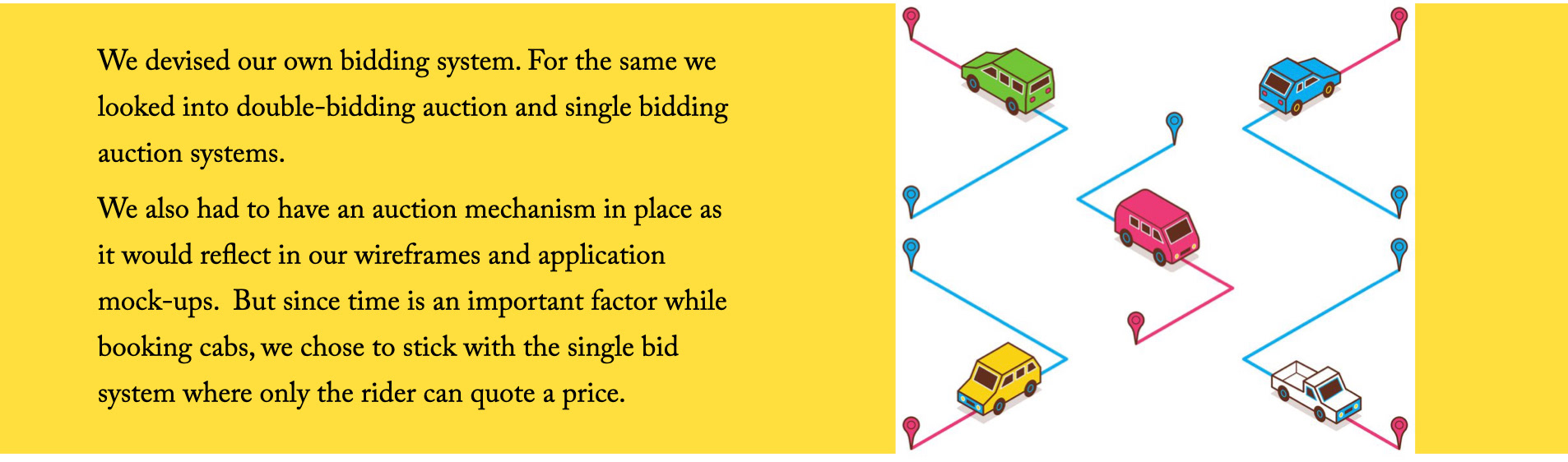
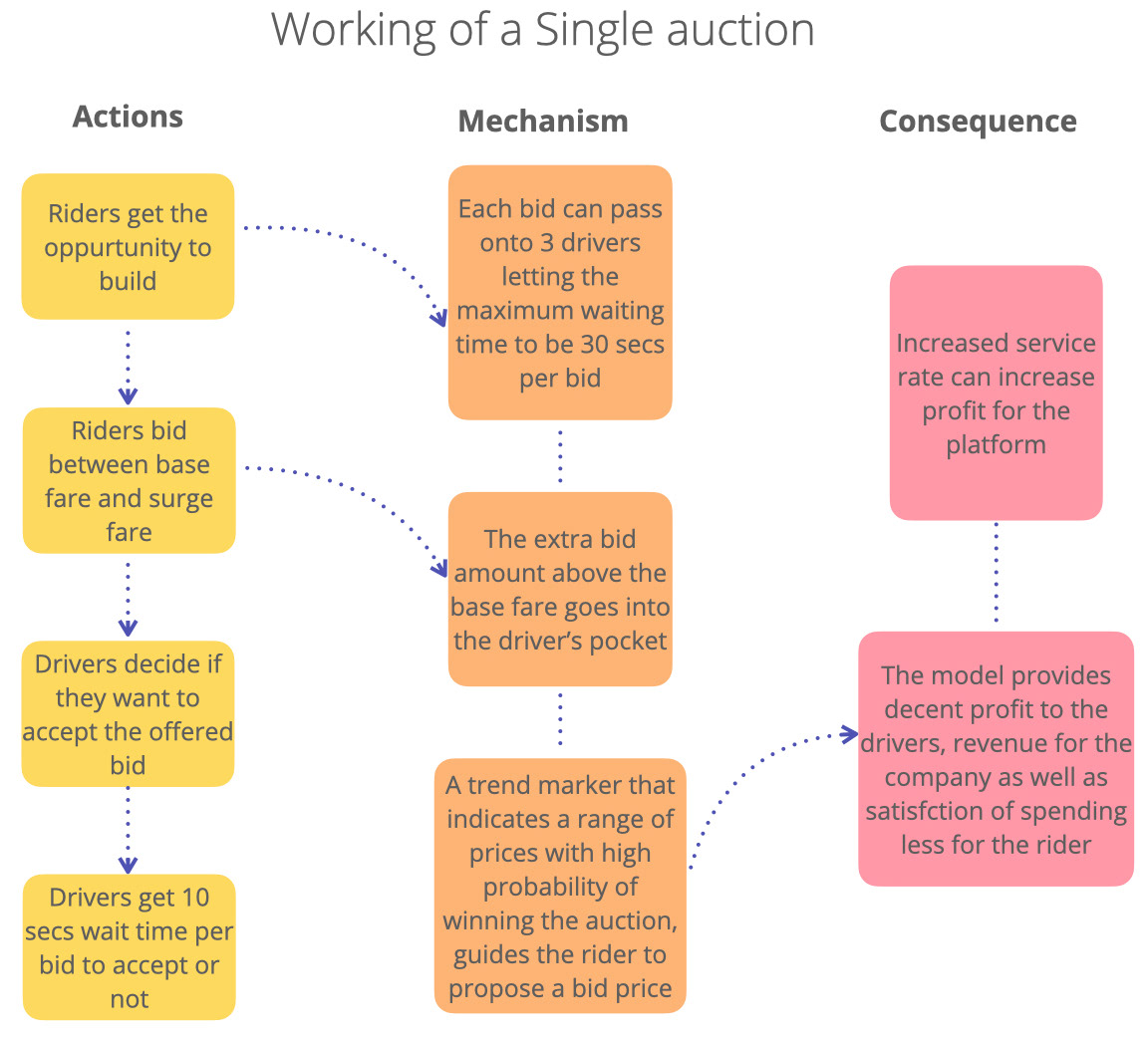
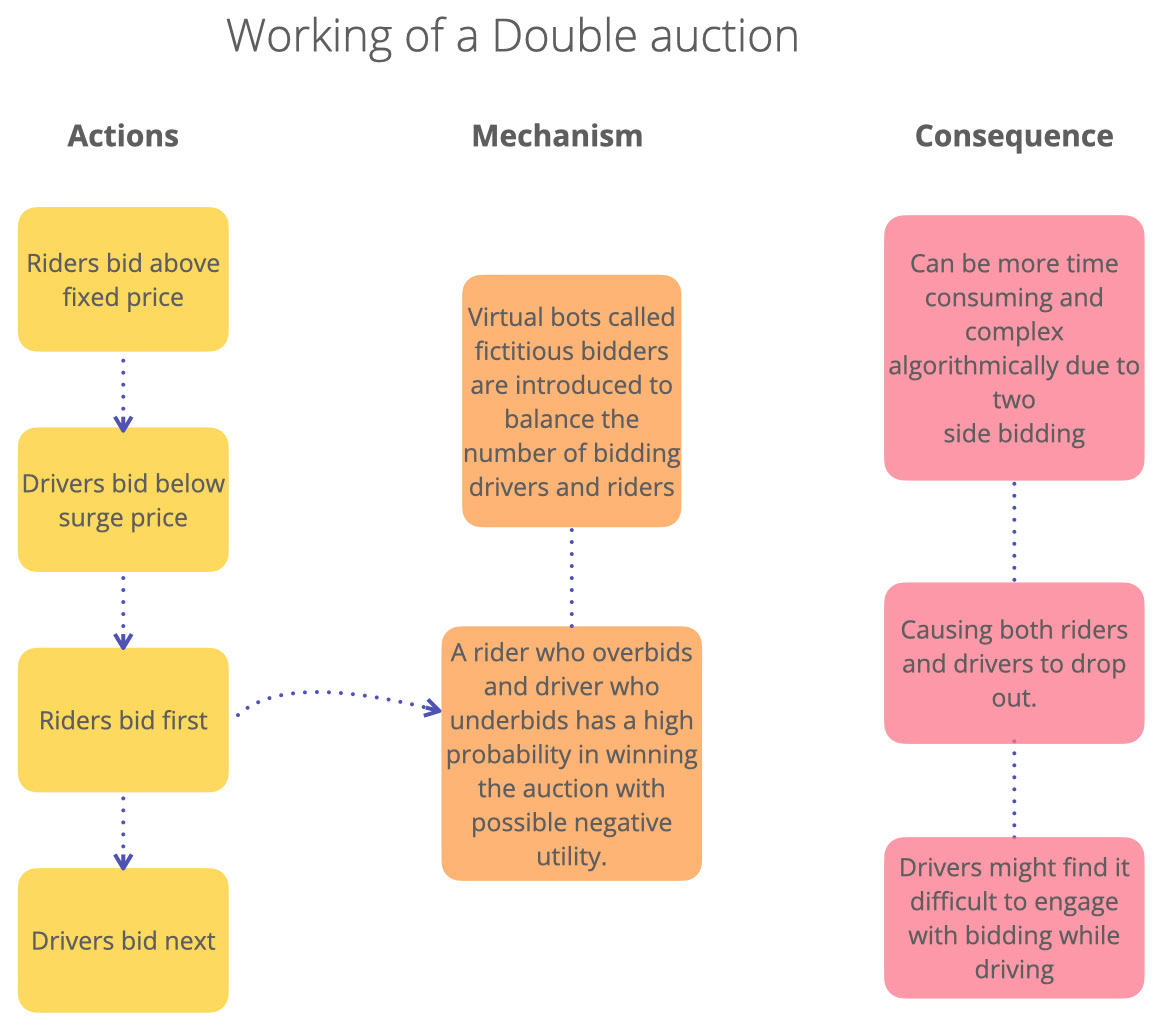
A short animation of proposed model for driver allocation for negotiation feature
Principles of the proposed mechanics
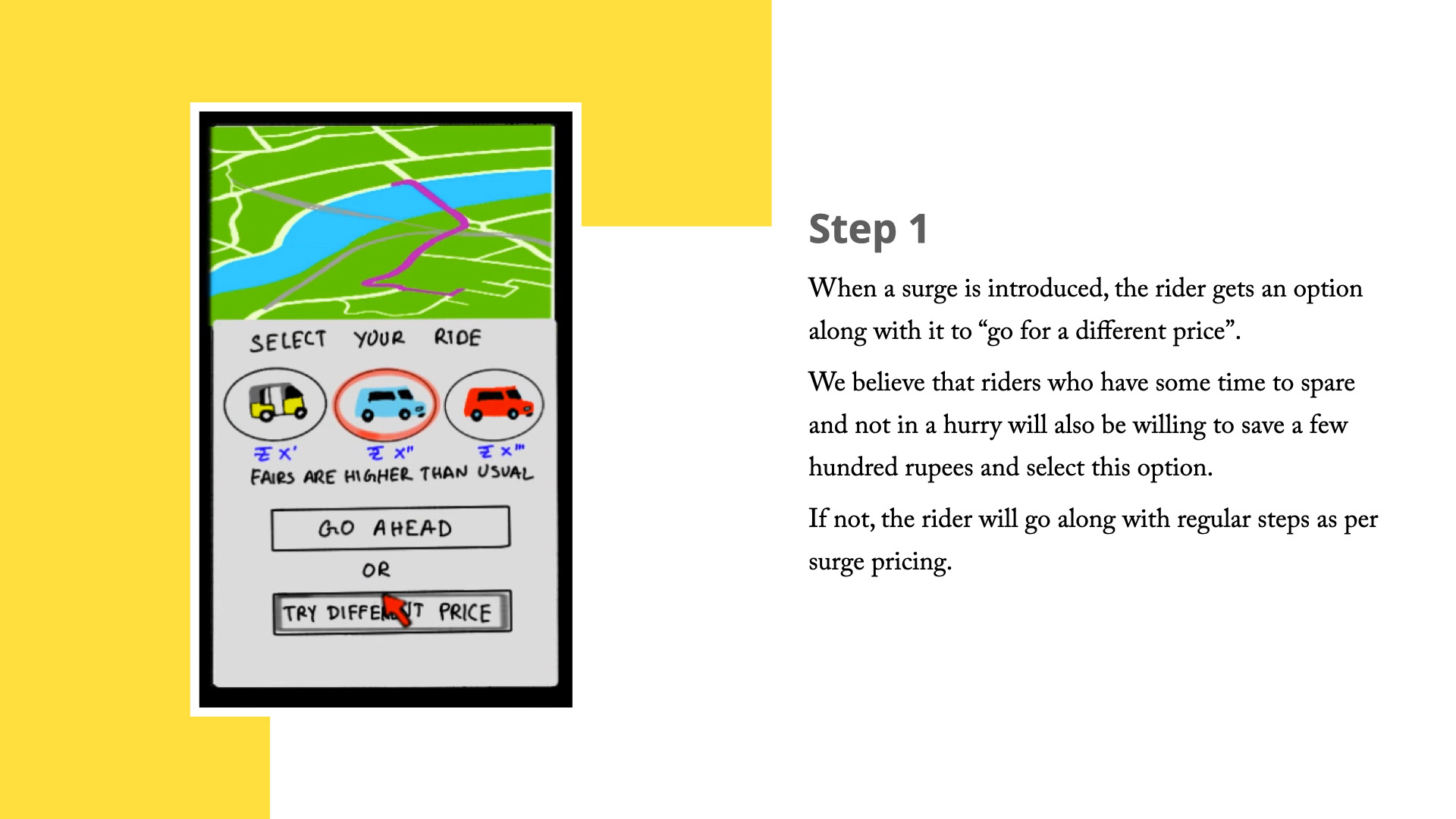
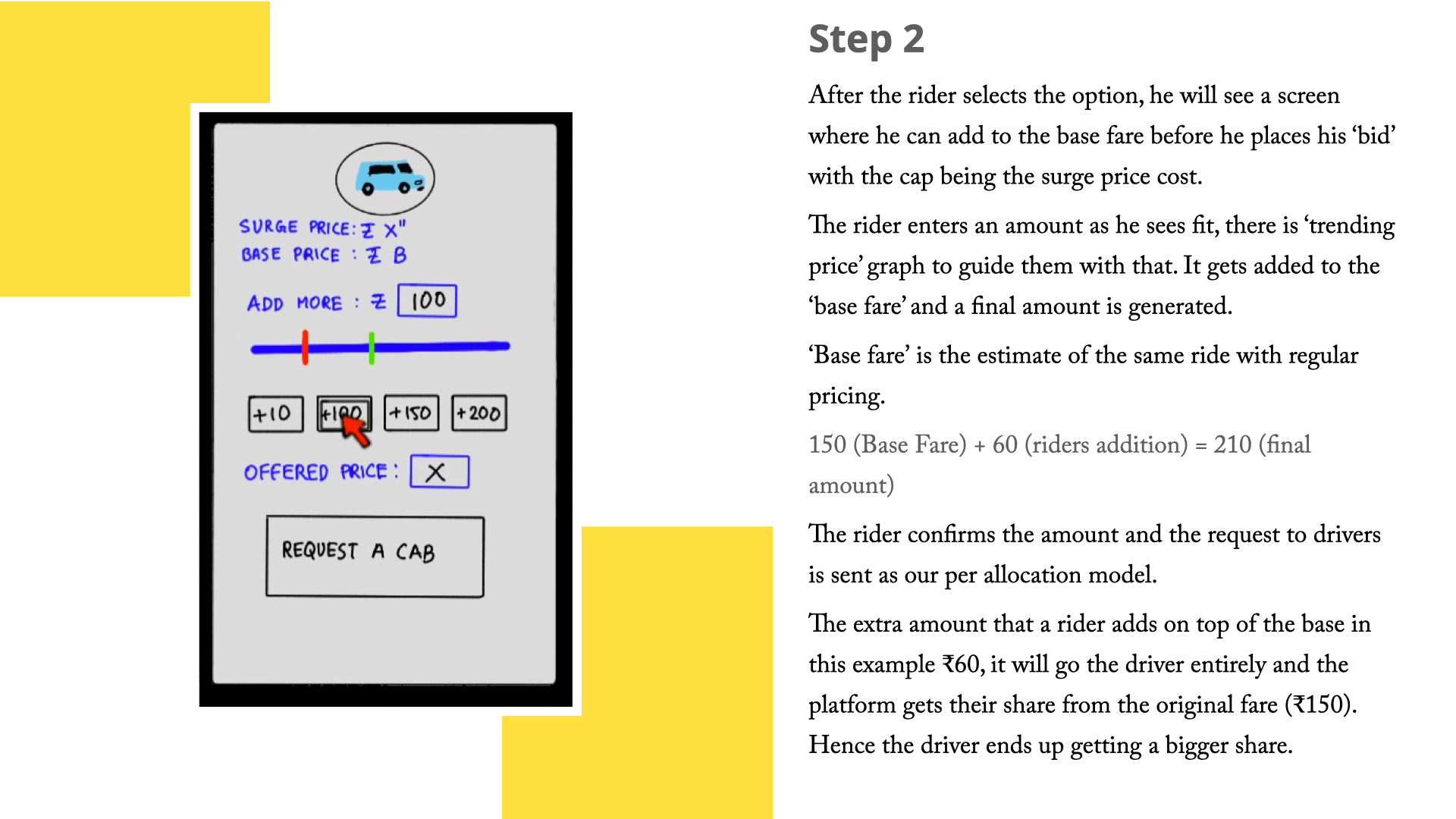
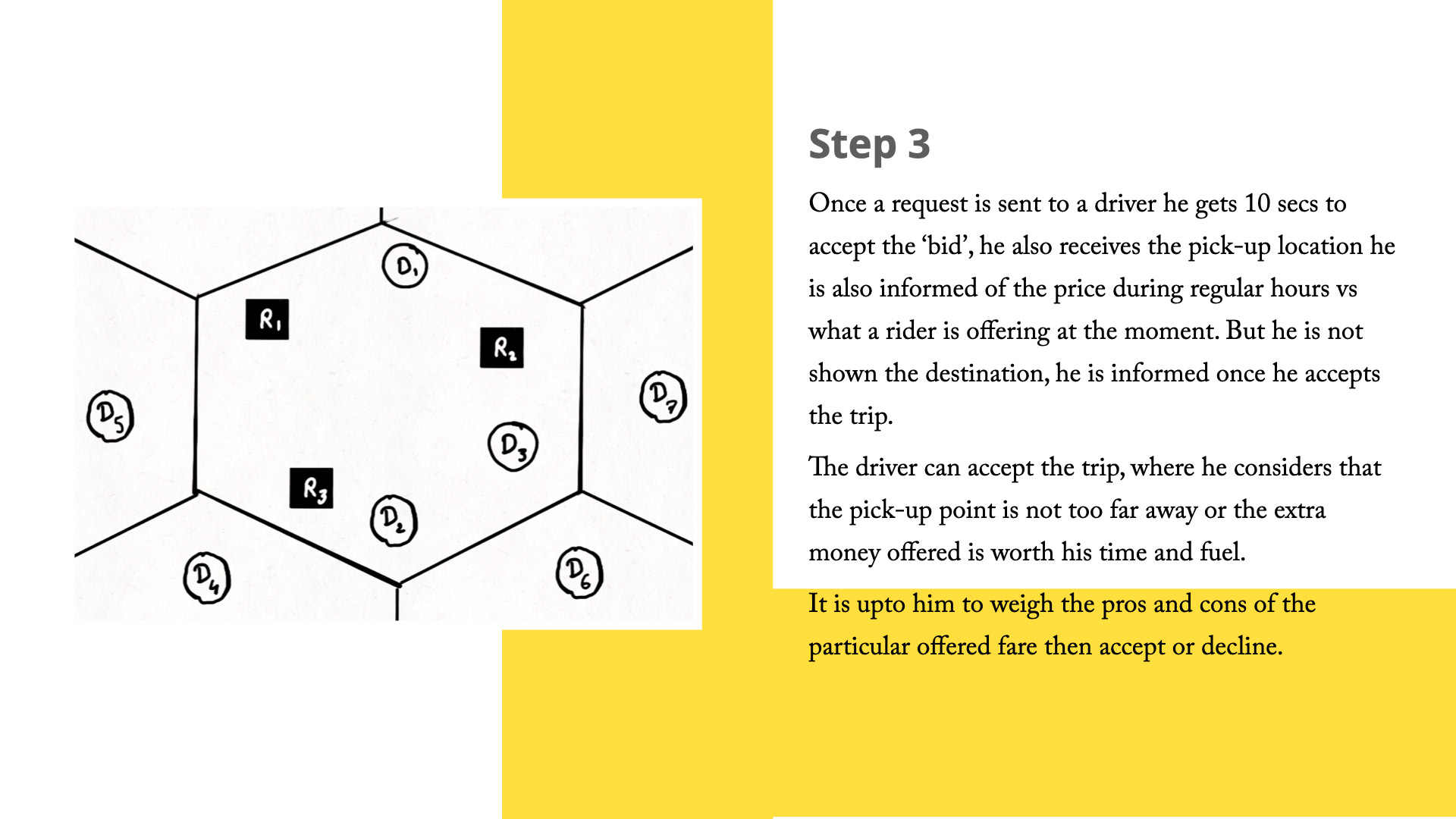
. . .
Application Mockup
We proposed this feature as a part of any existing ride hailing platform.
Screens we created are low fidelity examples of what it could be. Following are the rider’s screens.
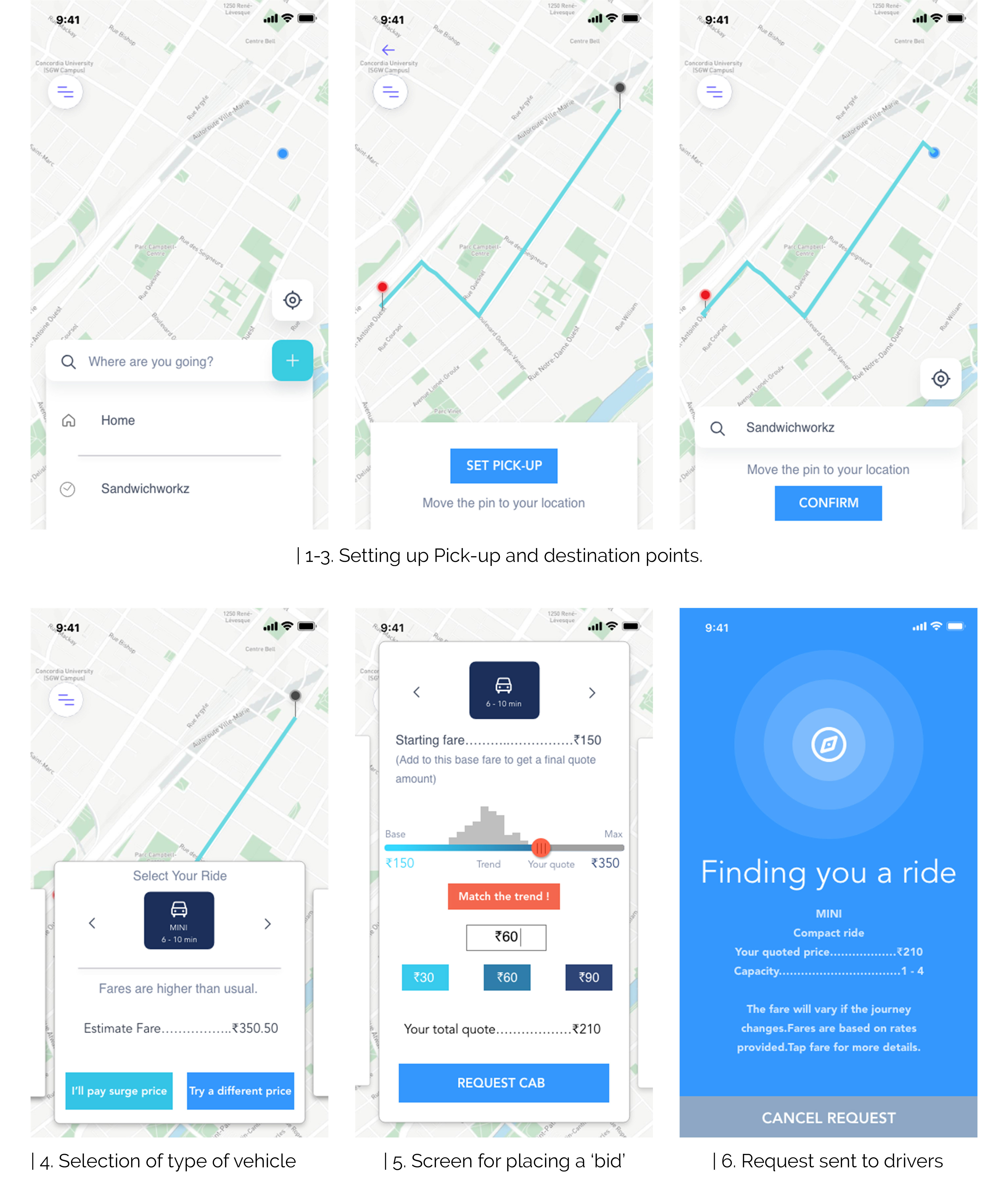
Futures wheel
We also realise that if this model gets implemented it might result in some ramifications in the future like taking a toll on the traffic and environment. We have explored a few of them in the Futures Wheel below.
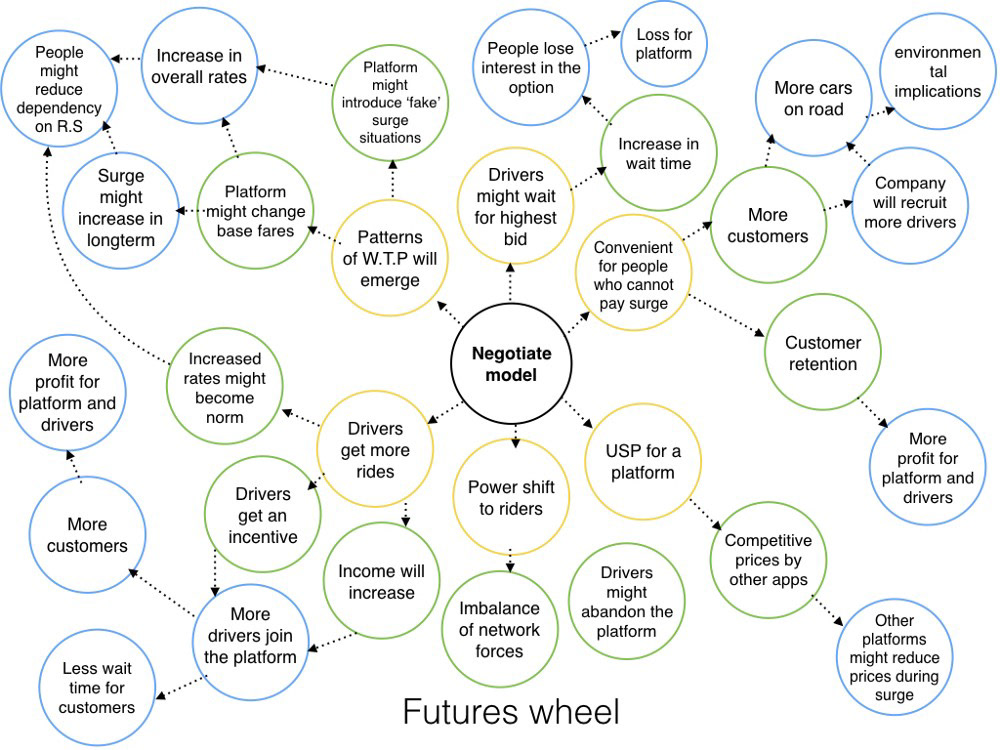
Learnings from this system of pricing
The working of this system completely depends on the efficiency of the algorithm. We have assumed a high level of efficiency for our simplicity sake with only proximity as a factor. But in the real world setting a number of variables like customer rating, driver rating, live traffic situation, time of day etc., which will be accounted for by the algorithm.
We also anticipate that this model if implemented for long could result in increased number of vehicles on road causing obvious problems. Also the platform is also exposed to vulnerabilities since since they are not controlling the price any longer. But we hope that it will atleast be a temporary solution to bridge the pricing disparity that exists.
Exploring Ride-sharing systems at scale (2018)
Duration of project : 5 weeks
Team - Meghana Upadhaya, Harinie Ananth,
Radhika Belkhede, Pritam Sarkar, Saili Gupte
...